Proximal Policy Optimisation for a Private Equity Recommitment System
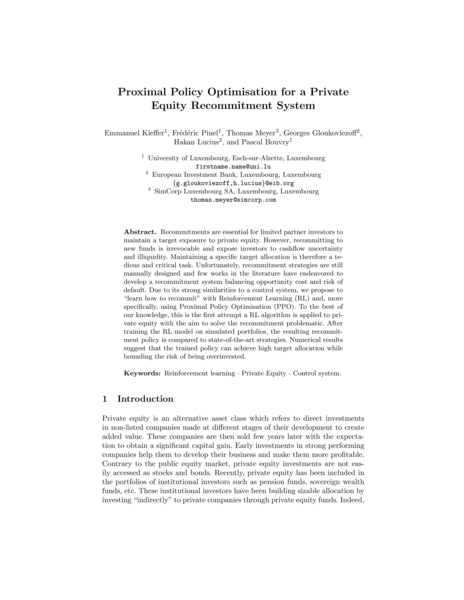
Abstract: Recommitments are essential for limited partner investors to maintain a target exposure to private equity. However, recommitting to new funds is irrevocable and expose investors to cashflow uncertainty and illiquidity. Maintaining a specific target allocation is therefore a tedious and critical task. Unfortunately, recommitment strategies are still manually designed and few works in the literature have endeavored to develop a recommitment system balancing opportunity cost and risk of default. Due to its strong similarities to a control system, we propose to “learn how to recommit” with Reinforcement Learning (RL) and, more specifically, using Proximal Policy Optimisation (PPO). To the best of our knowledge, this is the first attempt a RL algorithm is applied to private equity with the aim to solve the recommitment problematic. After training the RL model on simulated portfolios, the resulting recommitment policy is compared to state-of-the-art strategies. Numerical results suggest that the trained policy can achieve high target allocation while bounding the risk of being overinvested.
This work has been submitted to the Joint International Scientific Conferences on Artificial Intelligence (BNAIC/BNLEARN 2021).